Publications
Adaptive Phase-Field-FLIP for Very Large Scale Two-Phase Fluid Simulation

Capturing the visually compelling features of large-scale water phenomena,such as the spray clouds of crashing waves, stormy seas, or waterfalls, involves simulating not only the water but also the motion of the air interacting with it. However, current solutions in the visual effects industry still largely rely on single-phase solvers and non-physical “white-water” heuristics. To address these limitations, we present Phase-Field-FLIP (PF-FLIP), a hybrid Eulerian/Lagrangian method for the fully physics-based simulation of very large-scale, highly turbulent multiphase flows at high Reynolds numbers and high fluid density contrasts. PF-FLIP transports mass and momentum in a consistent, non-dissipative manner and, unlike most existing multiphase approaches, does not require a surface reconstruction step. Furthermore, we employ spatial adaptivity across all critical components of the simulation algorithm, including the pressure Poisson solver. We augment PF-FLIP with a dual multiresolution scheme that couples an efficient treeless adaptive grid with adaptive particles, along with a fast adaptive Poisson solver tailored for high-density-contrast multiphase flows. Our method enables the simulation of two-phase flow scenarios with a level of physical realism and detail previously unattainable in graphics, supporting billions of particles and adaptive 3D resolutions with thousands of grid cells per dimension on a single workstation.
Multiphysics Simulation Methods in Computer Graphics
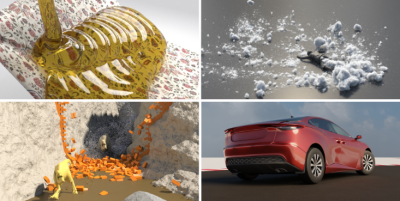
Physics simulation is a cornerstone of many computer graphics applications, ranging from video games and virtual reality to visual effects and computational design. The number of techniques for physically-based modeling and animation has thus skyrocketed over the past few decades, facilitating the simulation of a wide variety of materials and physical phenomena. This report captures the state-of-the-art of multiphysics simulation for computer graphics applications. Although a lot of work has focused on simulating individual phenomena, here we put an emphasis on methods developed by the computer graphics community for simulating various physical phenomena and materials, as well as the interactions between them. These include combinations of discretization schemes, mathematical modeling frameworks, and coupling techniques. For the most commonly used methods we provide an overview of the state-of-the-art and deliver valuable insights into the various approaches. A selection of software frameworks that offer out-of-the-box multiphysics modeling capabilities is also presented. Finally, we touch on emerging trends in physics-based animation that affect multiphysics simulation, including machine learning-based methods which have become increasingly popular in recent years.
@article{HJL*25,
journal = {Computer Graphics Forum},
title = {{Multiphysics Simulation Methods in Computer Graphics}},
author = {Holz, Daniel and Jeske, Stefan Rhys and L\"oschner, Fabian and Bender, Jan and Yang, Yin and Andrews, Sheldon},
year = {2025},
publisher = {The Eurographics Association and John Wiley & Sons Ltd.},
ISSN = {1467-8659},
DOI = {10.1111/cgf.70082},
volume = {44},
number = {2}
}
A Smoothed Particle Hydrodynamics framework for fluid simulation in robotics

Simulation is a core component of robotics workflows that can shed light on the complex interplay between a physical body, the environment and sensory feedback mechanisms in silico. To this goal several simulation methods, originating in rigid body dynamics and in continuum mechanics have been employed, enabling the simulation of a plethora of phenomena such as rigid/soft body dynamics, fluid dynamics, muscle simulation as well as sensor and actuator dynamics. The physics engines commonly employed in robotics simulation focus on rigid body dynamics, whereas continuum mechanics methods excel on the simulation of phenomena where deformation plays a crucial role, keeping the two fields relatively separate. Here, we propose a shift of paradigm that allows for the accurate simulation of fluids in interaction with rigid bodies within the same robotics simulation framework, based on the continuum mechanics-based Smoothed Particle Hydrodynamics method. The proposed framework is useful for simulations such as swimming robots with complex geometries, robots manipulating fluids and even robots emitting highly viscous materials such as the ones used for 3D printing. Scenarios like swimming on the surface, air-water transitions, locomotion on granular media can be natively simulated within the proposed framework. Firstly, we present the overall architecture of our framework and give examples of a concrete software implementation. We then verify our approach by presenting one of the first of its kind simulation of self-propelled swimming robots with a smooth particle hydrodynamics method and compare our simulations with real experiments. Finally, we propose a new category of simulations that would benefit from this approach and discuss ways that the sim-to-real gap could be further reduced.
@article{AAB+24,
title = {A smoothed particle hydrodynamics framework for fluid simulation in robotics},
journal = {Robotics and Autonomous Systems},
volume = {185},
year = {2025},
issn = {0921-8890},
doi = {https://doi.org/10.1016/j.robot.2024.104885},
url = {https://www.sciencedirect.com/science/article/pii/S0921889024002690},
author = {Emmanouil Angelidis and Jonathan Arreguit and Jan Bender and Patrick Berggold and Ziyuan Liu and Alois Knoll and Alessandro Crespi and Auke J. Ijspeert}
}
Previous Year (2024)